Optimizing Policy Targeting with Machine Learning: Evidence from Pakistani Audits
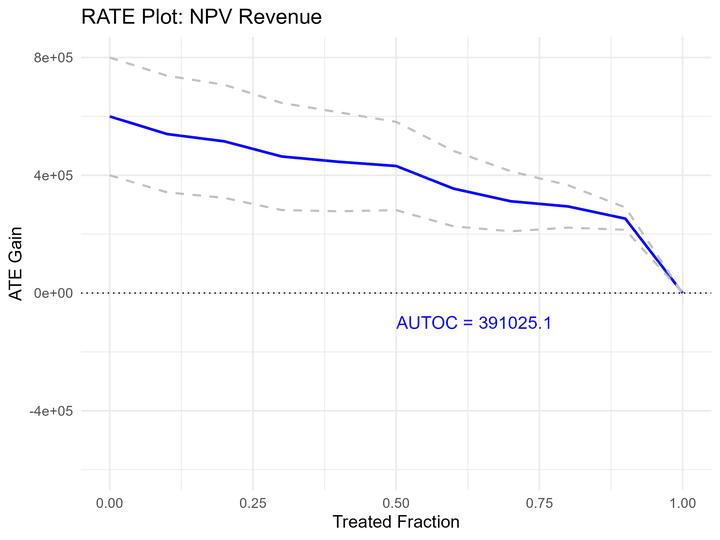
Abstract
We provide a framework for optimizing the distribution of policy treatment by combining machine learning techniques for the estimation of individualized causal responses with sufficient statistics for relative welfare implications of alternative distributions. This framework is applied to the setting of audit policy optimization in Pakistan. Specifically, we define a model which derives the Marginal Value of Public Funds (MVPF) in terms of three estimable causal effects of individuals in response to an audit – the net-present value of long-run tax liabilities, taxpayer burden from audit compliance, and government expenditures from the audit. With the universe of individual income tax returns in Pakistan from 2012โ 2020, we employ generalized random forests to estimate the individualized causal effects and optimize the distribution of audits with stochastic gradient descent and genetic algorithms. We find that the welfare cost per-dollar of revenue raised can be reduced by between 40%โ 57% while collecting even more revenue than under the observed policy.
Type